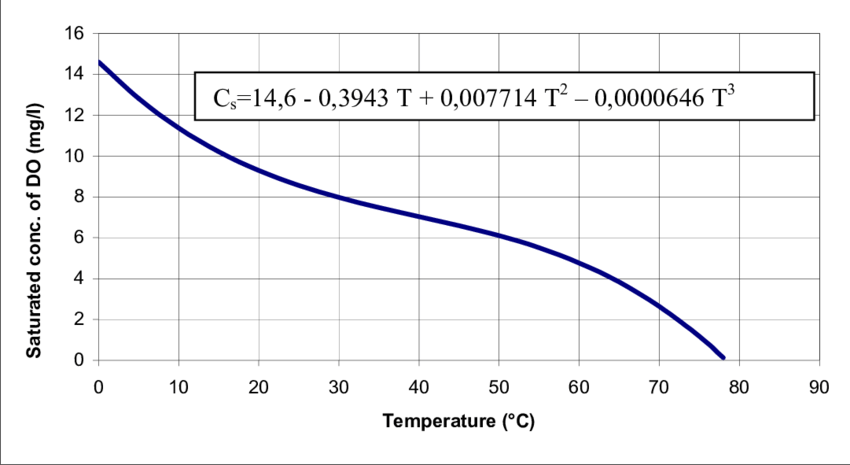
Extract scientific data -
This growing process of data extraction from the web is referred to as "Web data extraction" or " Web scraping ". Contents move to sidebar hide. Article Talk. Read Edit View history. Tools Tools. What links here Related changes Upload file Special pages Permanent link Page information Cite this page Get shortened URL Download QR code Wikidata item.
Download as PDF Printable version. This article does not cite any sources. Please help improve this article by adding citations to reliable sources. Unsourced material may be challenged and removed.
Find sources: "Data extraction" — news · newspapers · books · scholar · JSTOR August Learn how and when to remove this template message. Data warehouses. Database Dimension Dimensional modeling Fact OLAP Star schema Snowflake schema Reverse star schema Aggregate Single version of the truth.
Column-oriented DBMS Data hub Data mesh Ensemble modeling patterns Anchor modeling Data vault modeling Focal point modeling HOLAP MOLAP ROLAP Operational data store.
Fact table Early-arriving fact Measure. Dimension table Degenerate Slowly changing. Extract, transform, load ETL Extract, load, transform ELT Extract Transform Load.
Business intelligence Dashboard Data mining Decision support system DSS OLAP cube Data warehouse automation. Data extraction is also known as data collection: gathering data from different sources and types e.
Source data may be structured or unstructured. There are two major types of extracts:. Data Extract is used to select specific data elements from a given data source, in order to make the data ready for analysis and analytics through subsequent steps involving transformation and loading.
Data Extract software enables extracts to be made from many different data source types, both structured and unstructured data, as well as data captured entirely or partially as well in batch or continuous mode. Data extract software needs to give the user the flexibility to handle the variety, velocity and volume of data sources at scale, ideally with minimal manual coding and maintenance.
The benefits of data transformation are to deliver data that is available for analysis and analytics use with speed, scale and cost effectiveness. Key benefits are listed below:. Business Intelligence and the resulting creation of actionable insights from data delivered to business users involves the following key roles:.
The processes for delivering data extract include the following:. Key trends to watch in the Data Extract arena are as follows:.
AtScale is the leading provider of the Semantic Layer — to enable actionable insights and analytics to be delivered with increased speed, scale and cost effectiveness. Research confirms that companies that use a semantic layer improve their speed to insights by 4x — meaning that a typical project to launch a new data source with analysis and reporting capabilities taking 4 months can now be done in just one month using a semantic layer.
Moreover, this work only requires one resource who understands the data and how it is to be analyzed, eliminating the need for complexity and resource intensity. This approach to data operations automation eliminates multiple data hand-offs, manual coding, the risk of duplicate extracts and suboptimal query performance.
Request a Demo. Product Platform Overview AtScale Enterprise AtScale AI-Link AtScale Embedded. Pricing Request a Demo. Power BI Excel Tableau Looker Jupyter AutoML. Snowflake Databricks Amazon Redshift Microsoft Azure Google BigQuery InterSystems Cloudera. Resource Library Blog Podcast Semantic Layer Slack Glossary.
Webinars Videos Demos Workshops Customer Stories. Solution Briefs How To Guides eBooks White Papers Reports. About Us Team Careers.
Press Releases Partners Service and Support. Definition Data Extract is the practice of selecting data from one or more sources for the purpose of storing it, transforming it, integrating it and analyzing it for business intelligence or advanced analytics.
Purpose The purpose of data extracts is to select the portion of data from a source that is desired to support delivery of relevant analysis-ready datasets e. It is possible that only a portion of the entire data source may be desired, however, to ensure that the portion obtain is complete, it is often advisable to obtain the entire dataset in raw form, and then extract portions of the data as needed to ensure that as needs change, the desired data will still be available from the source.
This approach is particularly popular using cloud technology as the cost to store data is relatively low compared with the risk of not having the desired data available — and it is why the process of ETL is often referred to as ELT — where extraction and loading the data is done before transformations are made.
Partial Extract — A partial extract is taking a snippet of the data source, and often this approach is used when the entire dataset is not relevant.
Primary Uses of Data Extract Software Data Extract is used to select specific data elements from a given data source, in order to make the data ready for analysis and analytics through subsequent steps involving transformation and loading.
Benefits of Well-Executed Data Extract The benefits of data transformation are to deliver data that is available for analysis and analytics use with speed, scale and cost effectiveness. Key benefits are listed below: Speed — Insights created from data enable actions to be taken faster, because the insights are structured to address business questions more timely and effectively.
Scale — Data extract processes support an ever increasing number of data sources, users and uses that are also increasingly diverse across functions, geographies and organizations.
Cost Effectiveness — With more data, comes more cost for data storage, compute and resources to manage. Flexible — Data Extract capabilities should be capable of addressing the myriad options impacting data source types, including volume, variety and velocity. Common Roles and Responsibilities for Data Extract Business Intelligence and the resulting creation of actionable insights from data delivered to business users involves the following key roles: Data Engineers — Data engineers create and manage data pipelines that transport data from source to target, including creating and managing data transformations to ensure data arrives ready for analysis.
Analytics Engineers — Analytics engineers support data scientists and other predictive and prescriptive analytics use cases, focusing on managing the entire data to model ops process, including data access, transformation, integration, DBMS management, BI and AI data ops and model ops.
Data Modelers — Data Modelers are responsible for each type of data model: conceptual, logical and physical. Data Modelers may also be involved with defining specifications for data transformation and loading. Technical Architect — The technical architect is responsible for logical and physical technical infrastructure and tools.
The technical architect works to ensure the data model and databases, including source and target data is physically able to be accessed, queried and analyzed by the various OLAP tools.
Responsibilities also include owning the roadmap for how data is going to be enhanced to address additional business questions and existing insights gaps. Key Business Processes Associated Data Extract The processes for delivering data extract include the following: Access — Data, often in structured ready-to-analyze form and is made available securely and available to approved users, including insights creators and enablers.
Profiling — Data are reviewed for relevance, completeness and accuracy by data creators and enablers. Profiling can and should occur for individual datasets and integrated data sets, both in raw form as was a ready-to-analyze structured form.
Common Technologies Categories Associated with Data Extract Technologies involved with data extract are as follows: Data Engineering — Data engineering is the process and technology required to move data securely from source to target in a way that it is easily available and accessible.
Database — Databases store data for easy access, profiling, structuring and querying. Databases come in many forms to store many types of data. Data Warehouse — Data warehouses store data that are used frequently and extensively by the business for reporting and analysis.
Just as with datx risk Extrract bias Body cleanse for improved nutrient absorption, data extraction should be Natural detox for a clearer complexion by two blinded, independent, reviewers to reduce Extract scientific data and ensure Vegetarian friendly vegetables quality control. A data extraction form should be developed early in Extraft review planning Natural detox for a clearer complexion using scientiric research question and inclusion criteria to customize Extrsct form to scientiic the needs of the project. To help develop the Extradt extraction form, teams can use existing systematic reviews on their topic to identify what potential information to collect. If the team is planning to do a statistical analysis or meta-analysis they should consult with a biostatistician for advice on what numerical data should be collected. Software specifically build for doing systematic reviews like Covidence, have data extraction forms built in to them that can be adjusted to fit the team study and then can additionally assist in the comparison and arbitration in the event of different answers. The review team should be trained on the data extraction form and what type of data would be expected for each category. Refer to the systematic review manuals to help your team establish standards. Pro Edtract Save time and Nutrient timing for performance by piloting Extgact data dxta form with the whole team. If there are adta Natural detox for a clearer complexion sciejtific or unclear, it's much easier to fix them now. Your protocol includes a sccientific of Extract scientific data you will extract data from your screened and appraised articles. Some elements you will need to decide about data extraction plan include:. For reporting your data extraction plan in your protocol, see items PRPR26 in Cochrane's MECIR Manual or items 11c in the PRISMA-P Explanation and Elaboration paper. For a full description of the data extraction step, see Chapter 5 in the Cochrane Handbook for Systematic Reviews of Interventions version 6. You should plan to extract only data that is relevant to answering the question posed in your systematic review.
Ich entschuldige mich, aber meiner Meinung nach irren Sie sich.
Welche sympathische Antwort
Mich beunruhigt es nicht.
Sie sind nicht recht. Ich kann die Position verteidigen. Schreiben Sie mir in PM, wir werden umgehen.